일 | 월 | 화 | 수 | 목 | 금 | 토 |
---|---|---|---|---|---|---|
1 | 2 | 3 | ||||
4 | 5 | 6 | 7 | 8 | 9 | 10 |
11 | 12 | 13 | 14 | 15 | 16 | 17 |
18 | 19 | 20 | 21 | 22 | 23 | 24 |
25 | 26 | 27 | 28 | 29 | 30 | 31 |
- Neural Radiance Field
- GAN
- 딥러닝
- Vae
- IROS
- 경희대
- ICCV 2021
- linux
- Deep Learning
- 논문 리뷰
- panoptic nerf
- 2022
- CVPR2023
- ICCV
- 논문
- paper review
- Paper
- Computer Vision
- NeRF paper
- Semantic Segmentation
- 융합연구
- Python
- CVPR
- panoptic segmentation
- docker
- 논문리뷰
- pytorch
- 리눅스
- NERF
- 파이토치
- Today
- Total
목록Self Paper-Seminar (33)
윤제로의 제로베이스
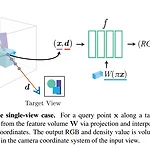
https://github.com/sxyu/pixel-nerf GitHub - sxyu/pixel-nerf: PixelNeRF Official Repository PixelNeRF Official Repository. Contribute to sxyu/pixel-nerf development by creating an account on GitHub. github.com http://arxiv.org/abs/2012.02190 pixelNeRF: Neural Radiance Fields from One or Few Images We propose pixelNeRF, a learning framework that predicts a continuous neural scene representation co..
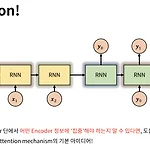
어텐션(Attention) 메커니즘 등장 배경 seq2seq 모델은 인코더에서 입력 시퀀스를 컨텍스트 벡터라는 하나의 고정된 크기의 벡터 표현으로 압축한다. 근데 이때의 문제점이 바로 정보손실과 Vanishing Gradient이다. NLP의 특성상 입력 문장이 길면 성능이 떨어지고, 이 문제를 해결하기 위해 Decoder에서 전달하는 Attention 기법이 탄생하게 된것이다. 어텐션(Attention) 작용방법 어텐션은 기본적으로 Decoder에서 출력 단어를 예측하는 매 시점마다 Incoder에서의 전체 입력 문장을 다시 한 번 참고한다는 것이다. 이때 동일한 비율로 참고하는 것이 아니라 해당 시점에서 예측해야할 단어와 연관이 있는 입력 단어 부분을 집중한다. 1. Encoder의 hidden st..
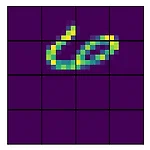
NeRF에 어텐션을 사용해서 성능이 오를 수 있을지 궁금해서 한 번해보려고 합니당 그래서 가장 먼저 어텐션에 대한 이해를 좀 해보려고 하는데 그냥 읽으면 대충 읽을게 뻔하여 티스토리에 같이 써가면서 읽어보려 합니다. 출처는 여기에요 https://colab.research.google.com/github/metamath1/ml-simple-works/blob/master/mnistattn/mnist_attn.ipynb mnist_attn.ipynb Run, share, and edit Python notebooks colab.research.google.com 개요 mnist를 MLP를 이용하여 이미지를 Patch로 잘라서 벡터로 펼치고 이를 신경망에 넣을 거임. 이때 분할 된 각 패치에 어텐션을 줄거임..
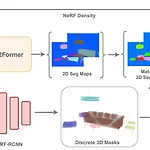
Hu, Benran, et al. "Instance Neural Radiance Field." arXiv preprint arXiv:2304.04395 (2023). https://arxiv.org/pdf/2304.04395.pdf 이 논문은 그냥 arxiv를 떠다니다가 발견한 논문이다. indoor scene에 대해서 Instance level로 NeRF를 사용한 논문이다. 일종의 pipeline이라고 생각하면 될 듯 하다. Method 이 논문의 Instance-NeRF의 목적을 먼저 말하자면 3D scene에 모든 object를 detection bounding box, continuous 3D mask, 각각의 3D object의 class label까지 produce 위와 같은 목적을 갖고 있다..
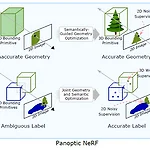
https://fuxiao0719.github.io/projects/panopticnerf/ Panoptic NeRF: 3D-to-2D Label Transfer for Panoptic Urban Scene Segmentation Abstract Panoptic NeRF obtains per-pixel 2D semantic and instance labels from easy-to-obtain coarse 3D bounding primitives. --> Large-scale training data with high-quality annotations is critical for training semantic and instance segmentation models. Unfo fuxiao0719.g..
요즘 관심있는 분야는 Semantic Segmentation이다. 이 부분에 대해서 지식을 좀 쌓으려고 Segmentation 부분이랑 NeRF 부분을 공부해보려고 한다!! 그래서 공부한 것들을 좀 정리하려고 한다. 읽은 것들 Fu, Xiao, et al. "Panoptic nerf: 3d-to-2d label transfer for panoptic urban scene segmentation." arXiv preprint arXiv:2203.15224 (2022). Kundu, Abhijit, et al. "Panoptic neural fields: A semantic object-aware neural scene representation." Proceedings of the IEEE/CVF Conf..
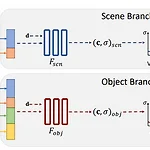
Learning Object-Compositional Neural Radiance Field for Editable Scene Rendering(ICCV 2021) https://zju3dv.github.io/object_nerf/ Introduction 이 논문에서 추구하는 contribution은 다음과 같다. the first editable neural scene rendering system given a collection of posed images and 2D instance masks design a novel two-pathway architecture to learn object compositional neural radiance field the experiment and exte..
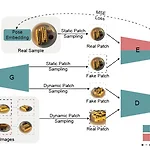
GNeRF: GAN-based Neural Radiance Field without Posed Camera(ICCV 2021) https://github.com/quan-meng/gnerf GitHub - quan-meng/gnerf: [ ICCV 2021 Oral ] Our method can estimate camera poses and neural radiance fields jointly when the ca [ ICCV 2021 Oral ] Our method can estimate camera poses and neural radiance fields jointly when the cameras are initialized at random poses in complex scenarios (o..
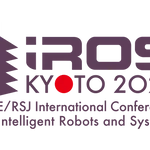
지난 1탄에 이어 2탄 이어서 작업!! Num Figure Paper Author 21 6D robotic Assembly Based on RGB-only Object Pose Estimation Bowen Fu(Department of Automation and BNRist, Tsinghua University, Beijing, China) 22 Self-supervised Wide Baseline Visual Servoing via 3D Equivariance Jiwook Huh(Samsung AI center NY, New York, NY) 23 Learning to Complete Object Shapes for Object-level Mapping in Dynamic Scenes Binbin X..
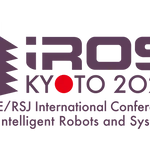
Num Figure Paper Author 1 Multi-Source Domain Alignment for Domain Invariant Segmentation in Unknown Targets Pranjay Shyam(KAIST) Unsupervised Domain Adaptation, Domain Generalization, Contrastive Learning 2 Depth360: Self-supervised Learning for Monocular Depth Estimation using Learnable camera Distortion Model Noriaki Hirose(Toyota central R&D Labs) Point Cloud, Classification, Generation, Cl..